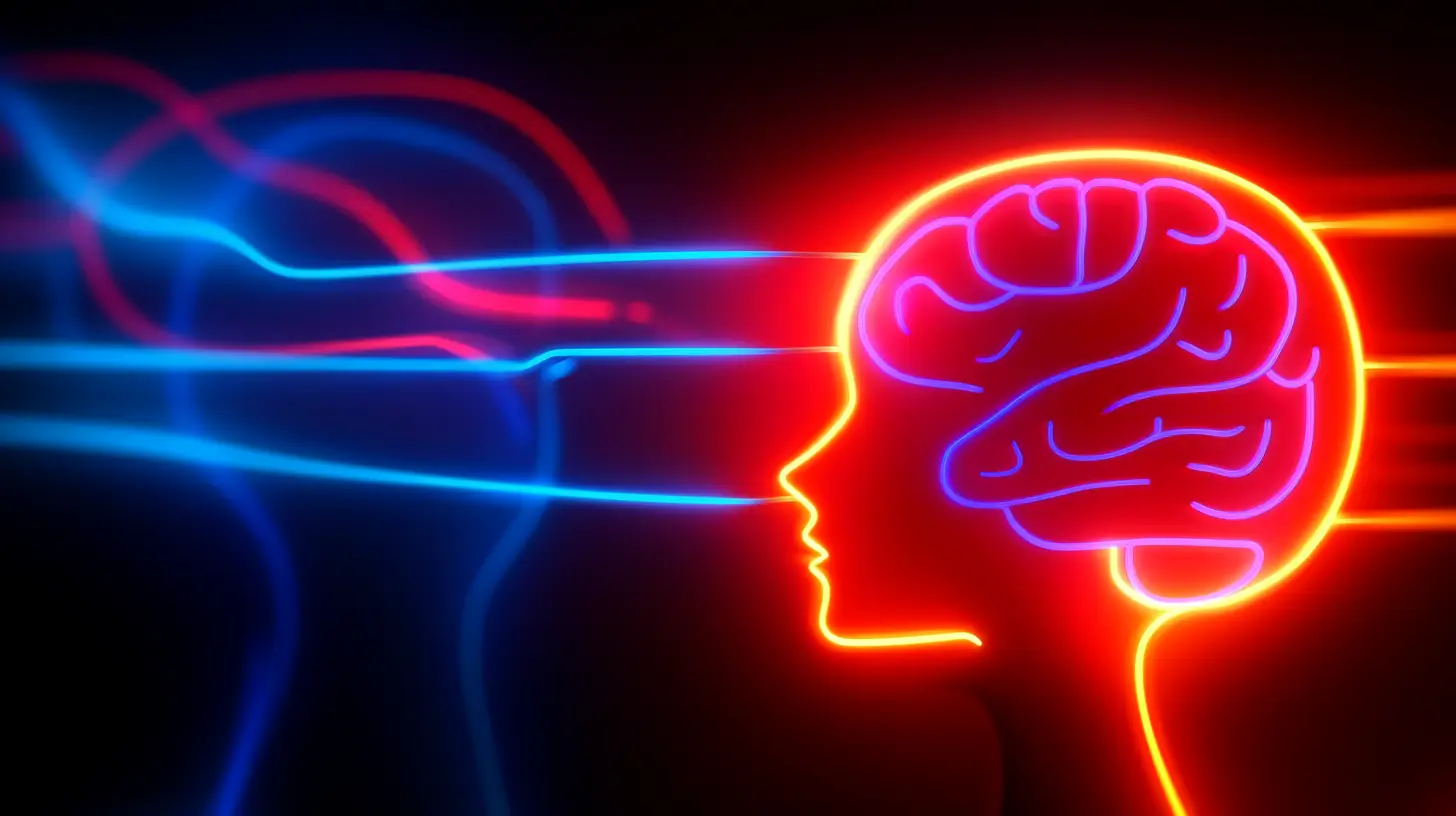
Customer Churn Prediction Model
The Challenge
HealthHive, a subscription-based health and wellness service, noticed a growing trend of customer churn, particularly among their most valuable subscribers. Without a clear understanding of the underlying causes or effective strategies to retain these customers, the company was losing revenue and market share.
Project Objectives
NeonFlow set out to achieve the following:
- Build a predictive model that could identify at-risk customers with over 85% accuracy.
- Provide actionable insights into the factors driving churn.
- Enable targeted retention campaigns to improve customer loyalty.
Crafting the Solution
The AI-powered churn prediction model analyzed customer behavior and engagement data to flag potential churn risks. Key features included:
- Behavioral Analytics: Monitored usage patterns, such as login frequency and feature engagement.
- Feedback Integration: Incorporated sentiment analysis from customer reviews and support interactions.
- Proactive Alerts: Sent real-time notifications about at-risk customers to the retention team.
Implementation Steps
- Data Collection: Aggregated customer data, including subscription history, engagement metrics, and feedback records.
- Model Development: Used supervised machine learning algorithms to identify churn indicators and predict risk levels.
- Retention Strategy Recommendations: Designed personalized offers, loyalty rewards, and targeted marketing campaigns based on model outputs.
Results Achieved
Within six months of implementation, the churn prediction model delivered:
- 30% reduction in overall churn rates.
- 15% increase in customer lifetime value (CLV).
- Enhanced retention of high-value subscribers through targeted interventions.
Client Feedback
HealthHive praised the solution as a “turning point” for their business, emphasizing its role in stabilizing their subscriber base and driving sustainable growth.
Lessons Learned
This project demonstrated the importance of understanding customer behavior and acting proactively to address dissatisfaction. Predictive analytics proved to be a powerful tool for fostering long-term loyalty.